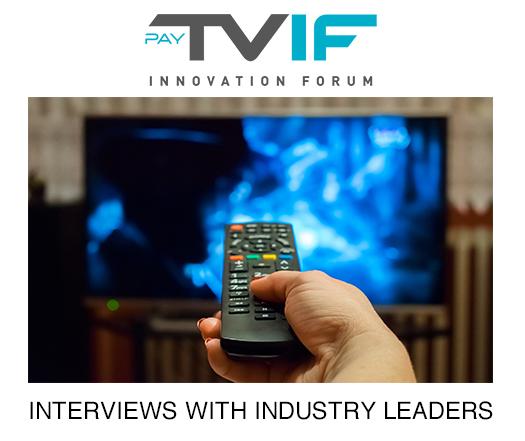
The Pay-TV Innovation Forum is a new global research programme for senior pay-TV executives, developed by NAGRA and designed to explore and catalyse innovation across the pay-TV industry, at a time of unprecedented change. As part of the programme, we are publishing a series of interviews with leading pay-TV industry executives from around the world to explore their views, perspectives and experiences of innovation in the pay-TV industry.
Pay-TV executives recognise the importance of advanced data and analytics, but agree that very few companies historically have had the scale and resources to harness it. However, there are strong signs that the pay-TV industry is likely to see significant developments in this area going forward: the 2017 Pay-TV Innovation Forum survey found that strong customer and market insight capabilities as well as big data and analytics solutions were among the top innovation priorities for pay-TV businesses in ensuring they are fit-for-the-future and well-positioned to innovate successfully.
In this latest interview, Chris Harvey, Director Group Strategy & Data at Foxtel, talks about the growing importance of advanced data and analytics in pay-TV and shares his advice for pay-TV providers who want to successfully implement advanced data and analytics in their businesses.
What are your key priorities in terms of data and analytics at Foxtel?
We are really focused on building the core data assets: we need to ensure that we have good data and that we can develop connections between different data sources to draw real insights about the behaviour of our customers, and be able to put the key pieces of information and insight in front of our management team, in the form of reports and dashboards, to inform their decisions.
With this in mind, one of the key priorities is to develop a rich insight into our customers’ viewing behaviour and to join the dots between viewing behaviour and other types of data we have access to. Once we understand how viewing behaviour can translate into churn or upsell opportunities, we can then leverage our data to grow the subscriber base and revenues we get from them. Another major priority is to build a targeted advertising proposition, where we can use data about our customers and their behaviour to serve one-to-one targeted advertising and promotions to them.
Do you think advanced data and analytics has become a real priority for the pay-TV industry in Australia?
It didn’t use to be as high on the priority list as it is should have been but that has changed in the last couple of years. Pay-TV businesses are very data rich in that there is not much more data they could be capturing that they are not already capturing. However, we have fallen behind in terms of the ability to join the dots on all that data and then develop insights from multiple data sources. Pay-TV businesses tend to have pockets of people who develop really interesting data analytics solutions for their specific business areas, but in terms of cross-functional capabilities – for example, understanding how viewing behaviour translates into churn or set-top box failures might trigger customer calls – there is still a lot that could be done. In order to create a single view of the customer we need to link different data sources, but we are still working on the overarching architecture that is able to do that with the aim of serving multiple business needs at once.
From your experience, do you have any practical advice for pay-TV providers who are trying to implement advanced data and analytics in their businesses?
I think the best way to do this is to start with small-scale and low-investment trials. This contrasts with the old ‘big bang’ approach of spending tens of millions of dollars building a large-scale data platform. This was often done without much trialling and therefore a lot of presumption around what would and would not work. As an example, let’s say you want to use insights into customers’ viewing behaviours to inform how they would be handled at a call centre. Ideally, call centre representatives would have access to a dashboard presenting all the relevant information and a set of recommended actions. One could invest a lot of money in developing this sort of a tool. Alternatively, you could pull this type of data together manually and test it to see whether it can deliver improvements before committing to a larger scale automated tool.
There are two important elements to consider here: firstly, how we can generate insight from the data we have and, secondly, how we can act on that insight to generate a beneficial outcome. The former is only about 20% of the job, whereas the latter is 80%, making it the difficult bit. For example, you might discover that proactively contacting customers may generate higher churn and that you have to develop more subtle ways of acting on signals in the data. However, you won’t know this until you test it. As a result, companies need to find low-cost ways of trialling various implementations before they commit to something they know will work for their business.
Which types of data are the most valuable for pay-TV providers?
Viewing data is definitely the most valuable piece of data that pay-TV providers have access to. Content is at the core of what we offer to our customers – the quantity and quality of what our customers are viewing are the key part of the utility we deliver. Having a deep understanding of this viewing behaviour helps pay-TV providers to manage their customers better and can potentially add value in other areas, such as valuing content or deciding which content to acquire. In addition, data on viewing behaviour is unique to pay-TV providers. Today, multiple other businesses can provide data on someone’s age, gender, location, income, but no one knows how that person spent three hours on Sunday afternoon watching TV. It is a very powerful strategic asset for pay-TV providers. This data obviously has significant value in advertising, but we will also see a host of other applications. Although pay-TV providers are quite guarded about their viewing data, I would like to believe that it could be monetised externally in other ways as well without giving away too much.
Which use cases for advanced data and analytics do you consider to be the most commercially attractive?
The most immediate commercial opportunities are in addressable TV advertising. However, in the longer term, the biggest benefits will most likely relate to subscriber revenues and content cost efficiencies, through improvements in marketing and content acquisition or commissioning. These will, however, take longer to realise.
How would you describe the vision in terms of advanced data analytics in pay-TV businesses?
I think this vision is constantly evolving. However, the starting point is to have your data set up so it is easy to access, easy to analyse in a joined up way and easy to share with anyone else in the business, with all of this aimed at delivering a single view of the customer and ideally enabling one-to-one relationships. Secondly, you need to have a strong analytics community within the organisation, which can continuously interrogate the data and try to find new ways of using it within the business. The analogy we sometimes use is that of a mining business where, on one hand, you’ve got well-established mining sites which are run business-as-usual and, on the other hand, you’ve got an exploratory team – equivalent to an analytics team – which is constantly looking for the next mining site. Once this exploratory or analytics team finds a new way to utilise insights through a series of trials, the business is ready to quickly incorporate it into the business-as-usual operations.